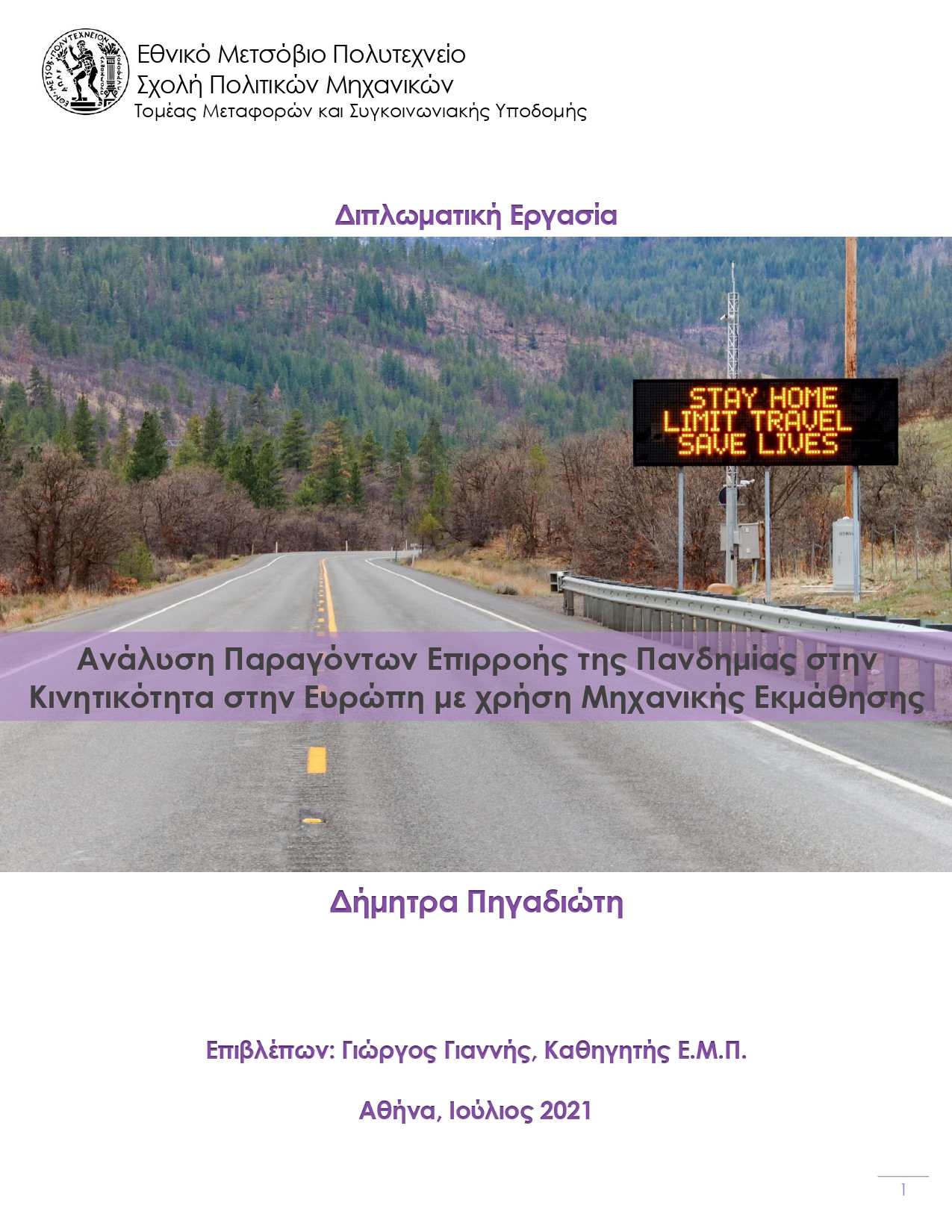
The aim of this Diploma Thesis is the analysis of pandemic impact factors in European mobility using machine learning. To achieve that, appropriate data has been collected regarding the tendency of people’s mobility, the mobility restrictions as well as the COVID-19 cases and deaths among twenty-six European countries. Data analysis was based on the development of appropriate regression analysis models, using machine learning techniques and making predictions, based on the historical trends of the dependent variables of driving and walking. Two types of models were developed. The basic difference of the models lies in the choice of the independent variables. The first one was based on the unique characteristics of each country and the factors that have the higher impact on mobility, while the second is a common data prediction model of the mobility of all study countries. The methodology demonstrated that the population’s behavior towards the pandemic and the consequent deaths, are better reflected and predicted by the characteristics of the population movements and not by the number of COVID-19 cases. Furthermore, the population’s mobility trends during the pandemic can be predicted satisfactorily for most European countries, considering the data of new COVID-19 cases per million population, the implemented vaccination policies and the movement trends to the grocery, pharmacy and parks. Moreover, it was demonstrated that the long-term implementation of the restrictive measures can lead to lower degrees of compliance.
ID | ad116 |
Presentation | |
Full Text | |
Tags | big data, driver behaviour, machine learning, naturalistic driving, statistical modelling, telematics |