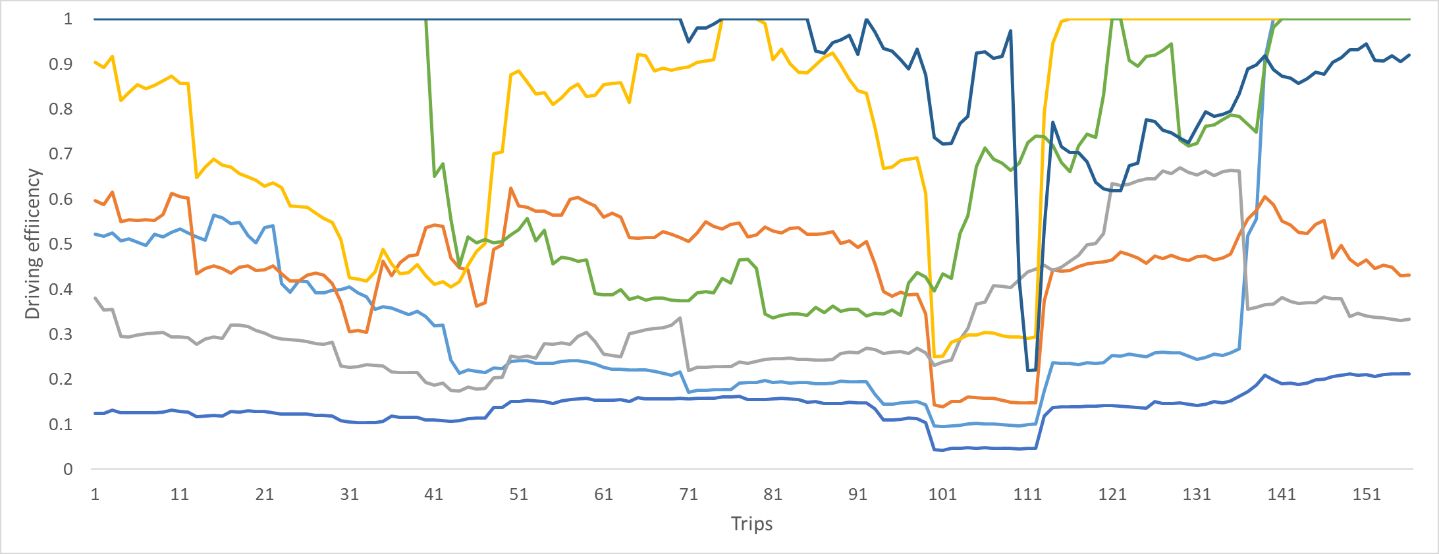
This paper attempts to shed light on the temporal evolution of driving safety efficiency with the aim to acquire useful insights for both drivers and road safety improvement. To this end, it presents a methodological framework to study the temporal evolution of measured driver’s efficiency with the aim to provide valuable information on the different driving behavior profiles.
Many studies in driving behavior literature (1, 2, 3, 4, 5) have focused on measuring driving safety efficiency. Nonetheless, only a few of them have demonstrated that there is a potential in analyzing and evaluating driving behaviour using microscopic driving data (e.g. driving over the speed limits, mobile phone usage and the number of harsh acceleration and braking events occurred while driving) collected from naturalistic driving experiments (1). As for data collection, literature review revealed that the methodologies most commonly used include driving simulators (6, 7), questionnaires (3) combined with simulators and naturalistic driving experiments (8, 9). Naturalistic experiments though provide a wide perspective of understanding typical microscopic travel and driving behaviour (10).
Driving efficiency assessment using microscopic driving parameters is thoroughly studied (1) but the evolution of the driving performance in time is not yet investigated. The temporal characteristics of driving efficiency and especially stationarity, trend and volatility, are of outmost importance when driving efficiency is measured. This is because the average driving efficiency might be representative of the total driving risk only in those cases when driving behavior is not fluctuating in time.
In this study, several statistical, econometric, optimization and machine learning techniques are exploited and applied on data collected from a sophisticated platform that uses smartphone device sensors during a naturalistic driving experiment. The driving groups arising are the a) typical drivers, b) unstable drivers and c) cautious drivers. This methodology could be exploited as a platform’s service in order to provide recommendations to drivers on how to improve their driving efficiency and become less risky.
ID | pc326 |
Presentation | |
Full Text | |
Tags | big data, driver behaviour, machine learning, naturalistic driving, statistical modelling, telematics |