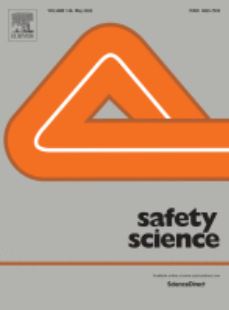
Harsh driving behavior events, such as harsh braking events (HBs) are road safety surrogate measures showing promising research venues towards crash mitigation, such as safety evaluations based on high-resolution driving data from smartphone sensors. This research presents a framework for aggregation and modelling of such data to highlight safety critical locations based on geometric and network characteristics. Spatial models including Geographically Weighted Poisson Regression, Bayesian Conditional autoregressive models (CAR), and variations of EXtreme Gradient Boosting (XGBoost) are implemented. The purpose is to: (i) explore parameters affecting frequencies of harsh driving events through causal spatial models in an urban road network and (ii) assess the predictive performance of models by testing the transferable components of these models in a new urban network test area. The models are trained and evaluated in terms of accuracy and transferability for HBs predictions in separate areas of Athens, Greece. Findings indicate that geometrical characteristics affect HB frequencies per road segment: Segment length and adjusted pass count are positively correlated with HBs, while gradient and neighborhood complexity are negatively correlated with HBs. Lane number and road type have more unclear and circumstantial effects overall. Two-lane segments have statistically higher HB frequencies compared to one-lane segments, while residential type segments have statistically lower HB frequencies compared to primary road segments. Furthermore, successful spatial predictions were conducted by averaging the results of all four methods, achieving accuracy of more than 87% for HB frequencies per road segment. Finally, the implications towards proactive traffic safety management and the extension possibilities for other harsh event types are also discussed.
ID | pj209 |
DOI | |
Tags | big data, driver behaviour, machine learning, naturalistic driving, statistical modelling, telematics |