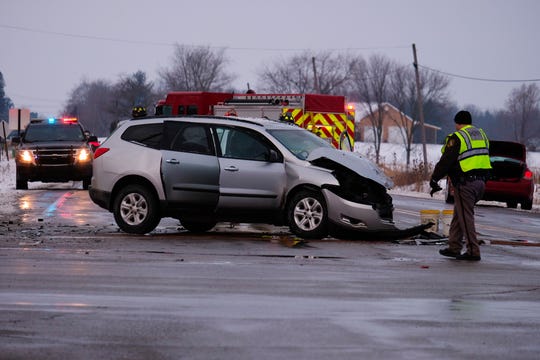
Crash occurrence analysis is the traditional approach to assessing traffic safety. Crash records can be erroneous or few, and so the analysis is not feasible in all cases. Unsafe traffic events such as near misses or harsh braking occur more frequently and can be easily obtained, e.g., by analyzing video data from an intersection. The present research aims to explore the relationship between crash frequency (i.e., average crashes per year) and the occurrence of two types of unsafe traffic events, namely near misses and harsh acceleration/ deceleration events, and assess whether crashes can be predicted based on unsafe traffic event data. Near misses will be obtained through video data for a given site, while acceleration/braking events will be obtained through smartphone app data across a network. It will be explored whether the type of traffic events as well as their spatiotemporal resolution (e.g., data from a weekday vs an eight-hour period) can improve the prediction of crashes at a given site (e.g., an intersection). Various egression models will be developed and assessed to conclude which combination of unsafe traffic events and spatiotemporal resolution yields the most accurate and reliable crash prediction models. The findings are expected to shift traffic safety analysis to more proactive approaches and indicate what are the minimum unsafe traffic event data requirements for traffic safety analysis.
ID | rn126 |
Name | UTE |
Duration | 2022-2024 |
Funding | National Technical University of Athens |
Research Institute | National Technical University of Athens |
Principal Investigator | Prof. G. Yannis |
Tags |