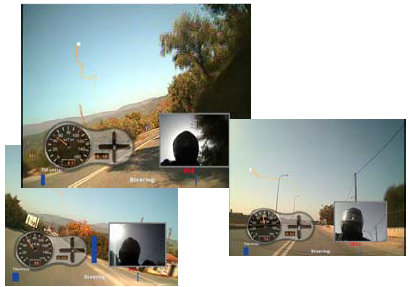
High resolution riding data from a large-scale Power Two Wheeler’s (PTW) naturalistic driving experiment are exploited in order to identify critical riding patterns emerging at the beginning and during an incident. A two-step analysis is adopted: first, a clustering approach is undertaken in order to reveal the critical rider’s actions at the beginning and during an incident. Second, the revealed actions are associated to specific riding situations in order to identify the critical riding patterns. Both methodological steps are modeled using Bayesian Networks. Results reveal three different prevailing riding actions for describing the onset of an incident and an equal number of actions that a rider executes during the course of an incident to avoid a crash. Furthermore, the proposed methodology efficiently relates the observed sets of actions with the different riding incidents and produce riding patterns (moving or stationary obstacle, overtaking and opposing traffic) that are characterized by different initial actions, as well as by different rider’s action likelihood during the incident.
ID | pc121 |
Presentation | |
Full Text | |
Tags | accident analysis, driver behaviour, motorcyclists |