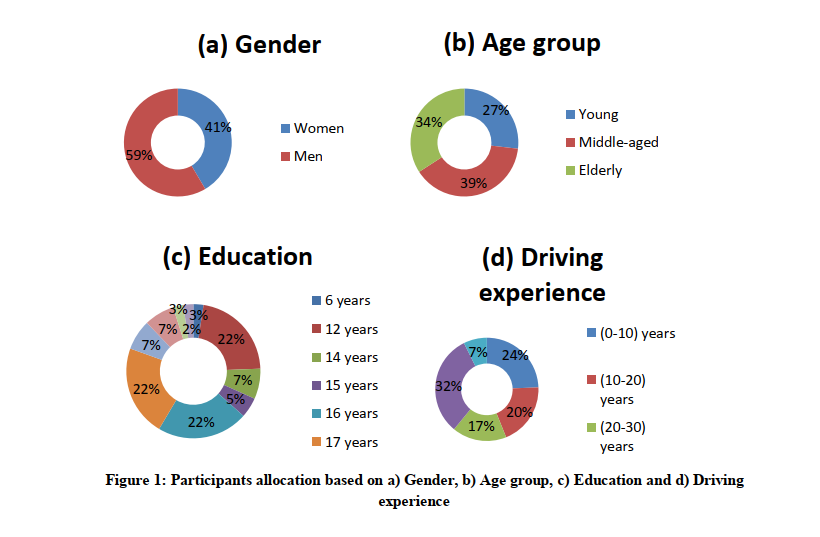
Background: The emergence of automated vehicles and new Advanced Driver Assistance Systems (ADAS), has brought about the need of identifying safety-critical events in real-time in urban environments. Nevertheless, the identification of critical factors contributing to such critical events is still ongoing. Objective: The aim of this paper is to determine critical factors for the identification of safety critical driving events in urban areas using highly disaggregated data from a driving simulator experiment. Method: Two statistical models were developed, namely a binomial logistic regression and a random forest one, in order to compare statistical and machine learning approaches for the identification of critical events. Furthermore, factor analysis was performed in order to investigate the existence of common factors in the group of independent variables. Results: The random forest model provided more reliable results in predicting events when compared to binomial logistic regression. More specifically, including more independent variables in the model was found to be more effective and with a lower false alarm rate. Moreover, factor analysis demonstrated that speed, the deviation of the vehicle from the middle of the road and the distance from the right boundary line can be event precursors, while speed and acceleration are representative variable during the event timeline. Conclusions: Speed as well as lateral and longitudinal acceleration along with lateral distances were found to be the most critical factors for identifying events in urban roads. Nevertheless, a larger sample of drivers and driving conditions (e.g. weather or distraction) and a naturalistic driving dataset could offer better results in future analyses.
ID | pc459 |
Presentation | |
Full Text | |
Tags |