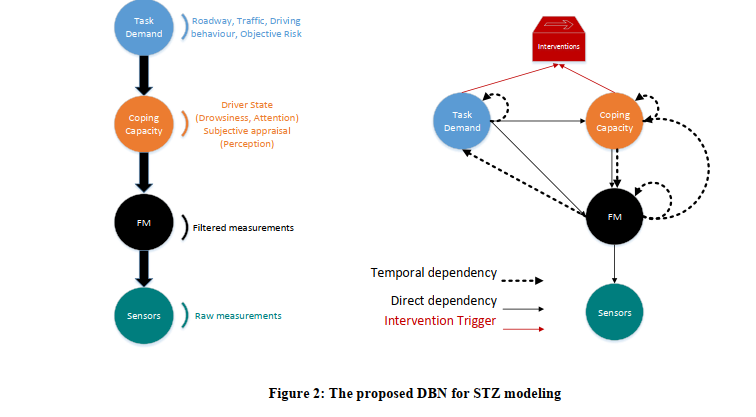
Within a transport system, a driver can be viewed as a (technology assisted) human operator, self-regulating control over a vehicle in the context of crash avoidance. Based on the Fuller’s task capability model, a Safety Tolerance Zone (STZ) concept has been recently developed in the i-DREAMS naturalistic driving study attempting to describe the point at which self-regulated control is considered safe. This paper aims to explicitly present the practical conceptualization of the STZ in order to transition from a theoretical framework to a practical implementation and a fully functional methodology. A thorough literature review of analytical models dealing with driver behavior and collision risk, both in real-time and post-trip, is first conducted and the most suitable modelling approaches for the STZ are selected. Specific machine learning algorithms and statistical models are then examined in order to relate driving performance with the probability of a rare event and the crash severityamong which, the most prominent approaches were initially found to be Dynamic Bayesian Networks (DBNs; a probabilistic graphical time-series model) and Long Short-Term Memory networks (LSTMs; a deep neural network formulation). Furthermore, Structural Equation Models (SEMs) and Discrete Choice Models (DCMs) were also deemed suitable for the i-DREAMS concept, providing ‘static’ or post-trip predictions, in contrast with DBNs and LSTMs which work dynamically (i.e. in real-time). For each of the aforementioned methods or techniques, a brief description of their underpinning procedure was presented, ollowed by their application for the identification of the STZ levels. The testing, calibration and enhancement of the mathematical models during the i-DREAMS simulation and onroad experiments can assure a sufficient and efficient data analysis, as well as timely initiation of the safety interventions.
ID | pc447 |
Presentation | |
Full Text | |
Tags |